Detecting novel lung cancer groups with Mapper
Published Paper DOI
10.1371/journal.pone.0284820

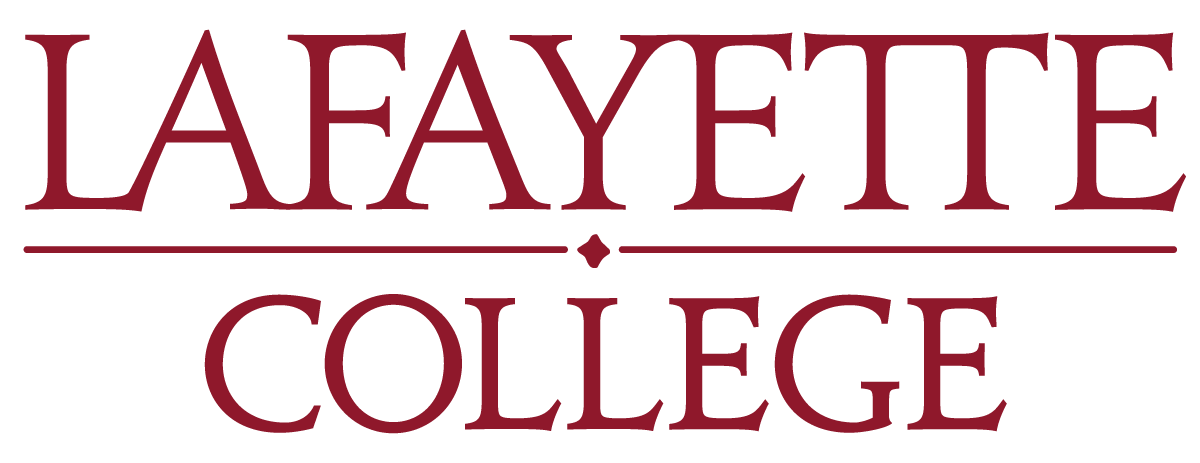
1. Division of Plant Sciences & Technology, University of Missouri, Columbia, MO
2. Department of Mathematics, University of Missouri, Columbia, MO
3. Department of Mathematics, University of Hawaii, Manoa, HI
4. Department of Mathematics, Lafayette College, Easton, PA
5. School of Life Sciences, University of Hawaii, Manoa, HI
This research was supported by National Institute of General Medical Sciences - Centers of Biomedical Research Excellence (COBRE) grant number P20GM125508.